By Wayne Gillam | UW ECE News
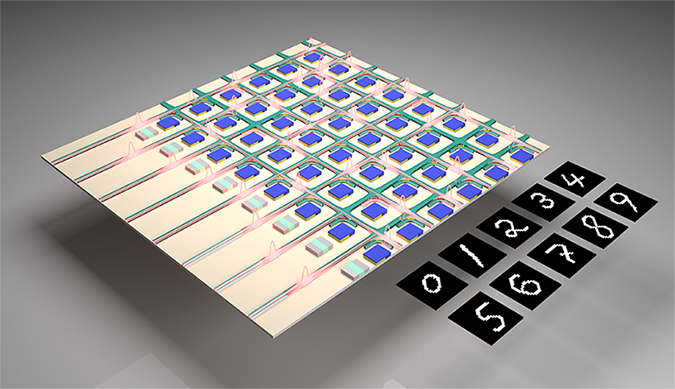
Artificial intelligence and machine learning are currently affecting our lives in a myriad of small but impactful ways. For example, AI and machine learning applications help to interpret voice commands given to our phones and electronic devices, such as Alexa, and recommend entertainment we might enjoy through services such as Netflix and Spotify. In the near future, it’s predicted that AI and machine learning will have an even larger impact on society through activities such as driving fully autonomous vehicles, enabling complex scientific research and facilitating medical discoveries.
But the computers used for AI and machine learning demand energy, and lots of it. Currently, the need for computing power related to these technologies is doubling roughly every three to four months. And cloud computing data centers used by AI and machine learning applications worldwide are already devouring more electrical power per year than some small countries. Knowing this, it’s easy to see that this level of energy consumption is unsustainable, and if left unchecked, will come with serious environmental consequences for us all.
UW ECE Professor Mo Li and graduate student Changming Wu have been working toward addressing this daunting challenge over the last couple of years, developing new optical computing hardware for AI and machine learning that is faster and much more energy efficient than conventional electronics. They have already engineered an optical computing system that uses laser light to transmit information and do computing by using phase-change material similar to what is in a CD or DVD-ROM to record data. Laser light transmits data much faster than electrical signals, and phase-change material can retain data using little to no energy. With these advantages, their optical computing system has proven to be much more energy efficient and over 10 times faster than comparable digital computers.
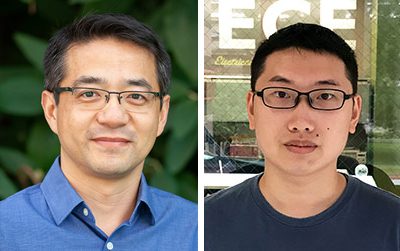
Now, Li and Wu are addressing another key challenge, the ‘noise’ inherent to optical computing itself. This noise essentially comes from stray light particles, photons, that interfere with computing precision. These errant photons come from the operation of lasers within the device and background thermal radiation. In a new paper published on Jan. 21 in Science Advances, Li, Wu and their research team demonstrate a first-of-its-kind optical computing system for AI and machine learning that not only mitigates this noise but actually uses some of it as input to help enhance the creative output of the artificial neural network within the system. This work resulted from an interdisciplinary collaboration of Li’s research group at the UW with computer scientists Yiran Chen and Xiaoxuan Yang at Duke University and material scientists Ichiro Takeuchi and Heshan Yu at the University of Maryland.
“We’ve built an optical computer that is faster than a conventional digital computer,” said Wu, who is the paper’s lead author. “And also, this optical computer can create new things based on random inputs generated from the optical noise that most researchers tried to evade.”
Using noise to enhance AI creativity
Artificial neural networks are bedrock technology for AI and machine learning. These networks function in many respects like the human brain, taking in and processing information from various inputs and generating useful outputs. In short, they are capable of learning.
In this research work, the team connected Li and Wu’s optical computing core to a special type of artificial neural network called a Generative Adversarial Network, or GAN, which has the capacity to creatively produce outputs. The team employed several different noise mitigation techniques, which included using some of the noise generated by the optical computing core to serve as random inputs for the GAN. The team found that this technique not only made the system more robust, but it also had the surprising effect of enhancing the network’s creativity, allowing it to generate outputs with more varying styles.
“This optical system represents a computer hardware architecture that can enhance the creativity of artificial neural networks used in AI and machine learning, but more importantly, it demonstrates the viability for this system at a large scale where noise and errors can be mitigated and even harnessed. AI applications are growing so fast that in the future, their energy consumption will be unsustainable. This technology has the potential to help reduce that energy consumption, making AI and machine learning environmentally sustainable and very fast, achieving higher performance overall.” — UW ECE Professor Mo Li
To experimentally test the image creation abilities of their device, the team assigned the GAN the task of learning how to handwrite the number “7” like a human. The optical computer could not simply print out the number according to a prescribed font. It had to learn the task much like a child would, by looking at visual samples of handwriting and practicing until it could write the number correctly. Of course, the optical computer didn’t have a human hand for writing, so its form of “handwriting” was to generate digital images that had a style similar to the samples it had studied but were not identical to them.
“Instead of training the network to read handwritten numbers, we trained the network to learn to write numbers, mimicking visual samples of handwriting that it was trained on,” Li said. “We, with the help of our computer science collaborators at Duke University, also showed that the GAN can mitigate the negative impact of the optical computing hardware noises by using a training algorithm that is robust to errors and noises. More than that, the network actually uses the noises as random input that is needed to generate output instances.”
After learning from handwritten samples of the number seven, which were from a standard AI-training image set, the GAN practiced writing “7” until it could do it successfully. Along the way, it developed its own, distinct writing style. The team was also able to get the device to write numbers from one to 10 in computer simulations.
As a result of this research, the team was able to show that an optical computing device could power a sophisticated form of artificial intelligence, and that the noise inherent to integrated optoelectronics was not a barrier, but in fact could be used to enhance AI creativity. They also showed that the technology in their device was scalable, and that it would be possible for it to be deployed widely, for instance, in cloud computing data centers worldwide.
Next steps for the research team will be to build their device at a larger scale using current semiconductor manufacturing technology. So, instead of constructing the next iteration of the device in a lab, the team plans to use an industrial semiconductor foundry to achieve wafer-scale technology. A larger scale device will further improve performance and allow the research team to do more complex tasks beyond handwriting generation such as creating artwork and even videos.
“This optical system represents a computer hardware architecture that can enhance the creativity of artificial neural networks used in AI and machine learning, but more importantly, it demonstrates the viability for this system at a large scale where noise and errors can be mitigated and even harnessed,” Li said. “AI applications are growing so fast that in the future, their energy consumption will be unsustainable. This technology has the potential to help reduce that energy consumption, making AI and machine learning environmentally sustainable — and very fast, achieving higher performance overall.”
This research is financially supported by the Office of Naval Research and the National Science Foundation. For more information, contact Mo Li.